ChatGPT, the new NLP (natural language recognition) model released by OpenAI on 1 December. Many people have just spent a happy and rewarding weekend with it.
The model is a derivative of OpenAI's NLP pre-training model, GPT-3, which will be released in 2020. Before this, it had been predicted that OpenAI would release GPT-4 at the end of this year or early next year. Although it is called GPT-3.5 instead of GPT-4,
this model has also been convinced important for the field of NLP as well as artificial intelligence.
ChatGPT is available for testing at: https://chat.openai.com/auth/login
As soon as ChatGPT was released, it was put up on the OpenAI website to be "tested" by the general public. The server for the free public beta version was quickly overwhelmed by enthusiastic beta testers. ChatGPT has been the subject of a lot of flirtation, discussion, questions, and chit-chat on social media.
How powerful is ChatGPT?
The GPT-3 is currently close to or even more capable than humans.
The ChatGPT model appears to be more potent than previous human-computer conversation models.
For example, it can question incorrect premises and assumptions and will actively admit mistakes. As well as the rejection of unanswerable and unreasonable questions, this shows a good understanding of the user's intentions and the accuracy of the results.
ChatGPT trains an initial model using supervised fine-tuning. AI-Based Humanlike Trainers provide the dialogue in which they play both sides - the user and the AI - and the AI trainers have access to the dialogue responses written by the model to help the AI adjust the content of the responses.
To create a reward model for reinforcement learning, the study needed to collect comparative data containing two or more model responses ranked by quality. The study collected conversations between AI trainers and chatbots and randomly selected a model-written message, extracted several alternative responses, and allowed the AI trainers to rank the responses. Besides, the study fine-tuned the model using a proximal policy optimization algorithm (PPO) and performed several iterations of the entire process.
Some users started having ChatGPT write novels. The whole process can be summarized as: humans control AI to write a story about how humans should get rid of AI in a world controlled by AI.
That’s interesting. Isn’t it?
The commercial implementation of ChatGPT
ChatGPT may give rise to a number of powerful NLP commercial applications. According to the AI industry's prediction, the popularity of general-purpose big models is expected to be achieved within 3-5 years, and AI will soon replace simple and repetitive work, even some process-oriented technical jobs, such as translation and newsletter editing. Generic Big Models are likely to change many ways of production and life now in a short period of time, with a large number of basic work processes being infiltrated or even replaced by intelligent applications based on Big Models.
So what are the challenges that ChatGPT, which represents the latest AI training trend, is likely to face in terms of commercialization?
Many industry insiders say that today's ChatGPT is still some way off from actually getting off the ground. One of the core issues lies in the accuracy of the model and the cost of deployment.
Firstly, ChatGPT's answers are not guaranteed to be accurate, which is fatal for business applications that need accurate answers to specific questions. This is the challenge ChatGPT needs to face if it is to be rolled out at scale on the C-suite. Some industry insiders worry that if AI outputs false information too quickly, it could drown out real information on the Internet and even mislead society as a whole.
This concern is not without merit, and is not without precedent, as Meta's earlier launch of Galactica, a large scientific prophecy model, was taken down after only three days because it answered questions too "out of control" and outputted a lot of false and fabricated content online.
ChatGPT is not immune to this problem, as John Schuman from OpenAI has said in previous interviews that they have made some progress in addressing the problem of AI fabrication, but not nearly enough.
Another issue for commercialization consideration is affordability. ChatGPT is currently in a free beta phase and the closest immediate practical application scenarios are search engine optimization, marketing media content creation assistance, and developer programming.
Sam Altman, CEO of OpenAI, has mentioned that one of the key updates to OpenAI in the future will be to provide Citations for ChatGPT-generated content.
With the number of GPT-3 participants reaching 175 billion, OpenAI is attempting to commercialize GPT-3 after its release in June 2020. OpenAI currently provides GPT-3 models to its developer customers for a fee in the form of an API, and charges based on the amount of token usage. Its customers include various fields such as media and marketing, and more than 300 apps have been generated based on GPT-3.
The high cost of the training also makes it difficult to quantify the value of AI applications from a commercial perspective in the early stages. As algorithm power increases, the number of scenarios increases, and the cost and energy consumption double, this will become a problem for all companies. Although OpenAI is currently valued at $20 billion, it has been argued that OpenAI should be a trillion-dollar company, but the company's main products and technologies are still in the experimental stage.
While many small-scale pre-trained models have had many successful commercial applications in today's human-machine conversations, large-scale models like ChatGPT are difficult to deploy in the To B space, with slow deployment, high costs and unclear commercial value, so they can only stay in applications such as search, culture, and entertainment.
The 2B industry requires more serious and rigorous AI. The current human-computer dialogue content is mainly focused on customer service, outbound calls, marketing, and other fields, which require a targeted model library and rapid training using smaller-scale models such as the UniLM framework based on BERT (pre-trained language representation model).
NLP, including ChatGPT, is still in an upward spiral in terms of commercialization, with a wide range of future applications, but the technology and business model need to find a "resonant" frequency as soon as possible.
However, there are many who believe that the future application areas of ChatGPT may not be limited to human-computer dialogue, but may extend to more applications, such as recognition of programming problems and search engines. But whatever the case, it will not be able to avoid those commercialization challenges.
The plight of artificial intelligence
AI industry is built on fundamental theory. The many waves that have happened to the AI industry have all eventually ebbed due to bottlenecks in theoretical development.
Another bottleneck is ethics. The development of technology is many times ahead of laws and regulations. In the study on Uber, Alex Rosenblat used the term "technological exceptionalism" to explain this phenomenon. When it comes to the ethics of artificial intelligence, most people think of serious issues such as liability for autonomous driving. Today, the technology of autonomous driving is ahead of the laws and regulations.
As the mature of generative AI, the copyright of AIGC and the issue of AI values are becoming big issues that limit the development of AI.
In the process of human-AI communication, how does AI learn, and can it output the correct values?
Large-scale training depends in part on the quality of the data, and AI cannot subjectively judge what is correct, so it is easy for AI to "learn the wrong things". Although ChatGPT has made great strides in 'gullibility', it can still be tempted to express less than 'politically correct views. This may be one of the obstacles to the commercialization of generic AI models.
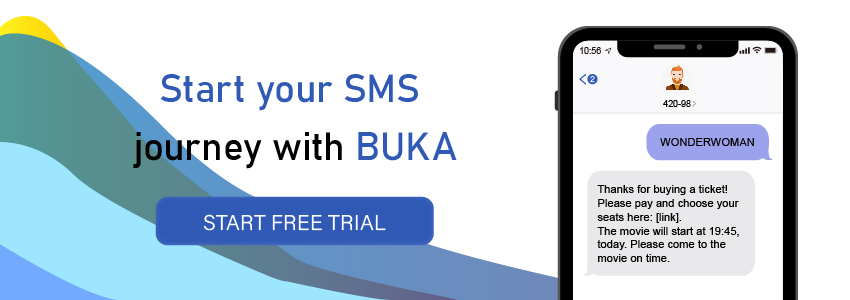